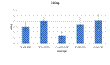

S[k]: Inverse
filter
G[k]: smoothed and spectrally
decimated version of the system measured transfer function.
ε:
regularization parameter. has been taken (typically) equal to 0.01
T[k] : target curve.
Marco Binelli1, Angelo Farina2
1 University
of Parma, Industrial Eng. Dept., Parco Area delle Scienze 181/A, 43100 PARMA,
ITALY
drumboogie@gmail.com
2 University of Parma, Industrial Eng. Dept., Parco Area delle Scienze 181/A, 43100 PARMA,
ITALY
farina@unipr.it
Digital equalization of
automotive sound systems
employing
spectral smoothed FIR filters
The usage of traditional
inversion techniques gives FIR filters longer or equal than the measured
impulse response. Because of the limited DSP computing power in automotive
field, we aim to reduce the filter length by spectral smoothing, as previously
observed by [1]. Other advantages of this method are a remarkable enlargement
of the sweet spot and the stability of the equalization
We developed a graphic Matlab1 function suite. It allows to plot the
measured frequency response and set all the filter parameters (length,
spectral resolution, target curve, regularization parameters)
1 Matlab is a registered trademark
of The MathWorks, Inc.
A blind listening test was
performed to investigate on subject’s filters liking. The 9 involved persons
were medium-high skilled. In detail, we chose some target curves and averaging
windows and asked the subjects to fill a questionnaire



The smoothing algorithm is the
same of [1]. It means that there is an independent computing for magnitude and
phase and this translates in a non-linear complex averaging
M: variable (magnitude or phase) to be smoothed
M’: smoothed variable
L: window length
α: half window length (L=2α+1)
W: window shape
Equation 1: magnitude/phase smoothing
The inversion technique is based on [3]. This ensures a
correct phase handling and absence of strong peaks in the filter spectrum. The
inverse filter S[k] can be computed as follow:
Equation 2: Inverse filter synthesis
We used some variable window
lengths rules: Critical Bands (CB), Equivalent Rectangular Bandwidths (ERB),
Double Octave Fraction (DOF) bands (1/24 octave below the car Schroeder
frequency, ≈ 800 Hz, 1/3 octave above).
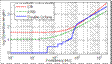
Figure 1: averaging window length vs frequency

Figure 2: magnitude plots shown after filter computation
Figure 3: magnitude of a frequency response
and target
curve
Figure 4: target curves used to build
the test filters
Figure 5: some measured spectra
of
test filters
Figure 6: evaluation questionnaire
(D,
E, F filter omitted)
From Student’s t test we can say
that the filters with “hard” target curve are the best between the tested
configurations. You can see this from the Student’s t test (raw A B, table 1)
and from the relationship between “spectral distance” and “liking” (figure 8).
It
is difficult to establish directly if there is an averaging window better than
another but it is possible to say that filters liking increases with the
smoothness of the spectrum (figure 9). Further investigations will be done on
smoothness types.
Other interesting results come from subjective parameters
relationships. We found 5 adjective well related to the global filters liking
(two are shown here, figure 10 and 11)
The resolution of the questions
scale was just 5 discrete steps, so it was hard to compare between average
values results because of the big standard deviation as you can see in figure
7.
Figure 7: “filter type-liking” histogram
with standard deviation indicators
Figure 8: “spectral distance - liking” relationship
Figure 9: “spectral roughness - liking” relationship
Table 1: Student’s t test on “Liking” parameter
Figure 10: “liking - bass balance” relationship
Figure 11: “liking - warmth” relationship

We tested inverse filters with 2
target curves (“Soft” and “Hard”) and 3 averaging windows (ERB, CB, DOF). Over
these, the native car sound configuration (not filtered) was inserted inside
the listening test.
This is the test filter set:
A –
Soft + ERB;
B –
Hard + ERB;
C –
Native (not filtered);
D –
Soft + DOF;
E –
Hard + CB.
This work was supported by:
AES 125 Convention
Paper 7575


In this paper we investigate
about the usage of spectral smoothed FIR filters for equalizing a car audio
system. The target is also to build short filters that can be processed on DSP
processors with limited computing power. The inversion algorithm is based on
the Nelson-Kirkeby method and on independent phase and magnitude smoothing, by
means of a continuous phase method as Panzer and Ferekidis showed. The filter
is aimed to create a "target" frequency response, not necessarily
flat, employing a little number of taps and maintaining good performances
everywhere inside the car's cockpit. As shown also by listening tests,
smoothness and the choice of the right frequency response increase the
performances of the car audio systems.